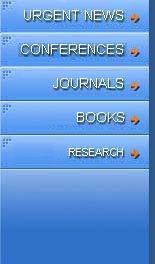 |
Main Page of the
Journal
Main Page of the WSEAS
Dec.2006, Jan. 2007,
Feb. 2007,
Mar. 2007, Apr. 2007,
May 2007,
Jun. 2007,
Jul. 2007,
Aug. 2007,
Sep. 2007
,
October 2007, Nov. 2007, Dec. 2007, 2008
WSEAS TRANSACTIONS
on
BIOLOGY and BIOMEDICINE
Issue 7, Volume 4, July 2007
Print
ISSN: 1109-9518
E-ISSN: 2224-2902 |
|
|
|
Title of the Paper: Fuzzy Possibility C-Mean Based on Mahalanobis Distance and Separable Criterion
DOWNLOAD FULL PDF
Authors: Hsiang-Chuan Liu, Der-Bang Wu,
Jeng-Ming Yih, Shin-Wu Liu,
Abstract: The well known fuzzy partition clustering algorithms are most based on Euclidean distance function, which can only be used to detect spherical structural clusters. Gustafson-Kessel (GK) clustering algorithm and Gath-Geva (GG) clustering algorithm, were developed to detect non-spherical structural clusters, but both of them based on semi-supervised Mahalanobis distance needed additional prior information. An improved Fuzzy C-Mean algorithm based on unsupervised Mahalanobis distance, FCM-M, was proposed by our previous work, but it didn’t consider the relationships between cluster centers in the objective function. In this paper, we proposed an improved Fuzzy C-Mean algorithm, FPCM-MS, which is not only based on unsupervised Mahalanobis distance, but also considering the relationships between cluster centers, and the relationships between the center of all points and the cluster centers in the objective function, the singular and the initial values problems were also solved. A real data set was applied to prove that the performance of the FPCM-MS algorithm gave more accurate clustering results than the FCM and FCM-M methods, and the ratio method which is proposed by us is the better of the two methods for selecting the initial values.
Keywords: FPCM-MS; FPCM-M, FCM-M; GK algorithms; GG algorithms; Mahalanobis distance
Title of the Paper: Fuzzy Clustering with New Separable Criterion
DOWNLOAD FULL PDF
Authors: Hsiang-Chuan Liu, Der-Bang Wu, Hsiu-Lan Ma
Abstract: Fuzzy clustering has been used widely in education, statistics, engineering, communication…etc. The fuzzy partition clustering algorithms are most based on Euclidean distance function, which can only be used to detect spherical structural clusters. Extending Euclidean distance to Mahalanobis distance, Gustafson-Kessel (GK) clustering algorithm and Gath-Geva (GG) clustering algorithm were developed to detect non-spherical structural clusters, but these two algorithms fail to consider the relationships between cluster centers in the objective function. Yin-Tang-Sun-Sun (YTSS) clustering algorithm solved the relationships between cluster centers question, unfortunately, they did not consider the distance between the center of all data points and the center of each cluster. This problem was solved and presented in this paper.
In this paper, a new fuzzy clustering algorithm (LWM) was developed based on the conventional fuzzy c-means (FCM) to obtain better quality clustering results with new separable criterion and better initial value. It is different from YTSS cluster algorithm. The improved equations for the membership and the cluster center were derived from the alternating optimization algorithm. Ten fuzzy scattering matrices in the objective function assure the compactness between data points and cluster centers, and also strengthen the separation between cluster centers in terms of a new separable criterion. The conclusions were drawn from this study as follows. (a) The distance between the center of all points and the center of each cluster was considered to obtain more accurate clustering results. (b) The singular problem was solved by using LWM algorithm. (c) The β value would not be required. The β value could be replaced by the best value, produced by the new LWM algorithm, developed by the authors of this paper. (d) Numerical data show that the LWM clustering algorithm gave more accurate clustering results than the FCM and YTSS clustering algorithm.
Keywords: GG clustering algorithm, GK clustering algorithm, YTSS clustering algorithm.
|
|
|